In our first event focussing on Higher Education and Artificial Intelligence, Lal Tawney, Whitecap’s Practice Lead for Education, hosted a discussion to explore the impacts of the pandemic on student progression, and how Artificial Intelligence could be best deployed in this space. The roundtable was attended by a broad mix: staff from UK universities including Deputy Vice-Chancellor, Heads of Faculty or Centre, Dean, Deputy Dean, Senior Lecturers, as well as the Managing Director of an Online Program Manager, and Director of an AI Assurance organisation.
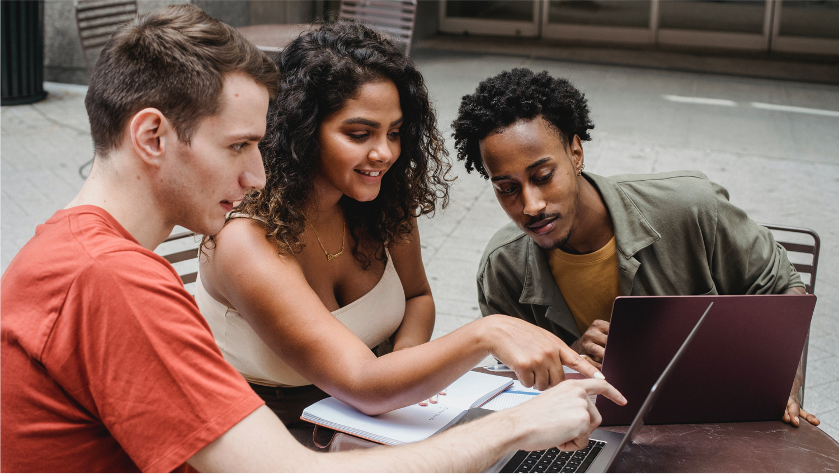
The Context
There are more students going to university – the number of first year higher education UK student enrolments has grown from c495,000 in 12/13 to c585,000 in 18/19.
The rates of student attainment have been declining – the projected outcomes of UK domiciled full-time first degree starters obtaining a degree was 81.8% for those starting in 12/13 vs 79.4% starting in 17/18.
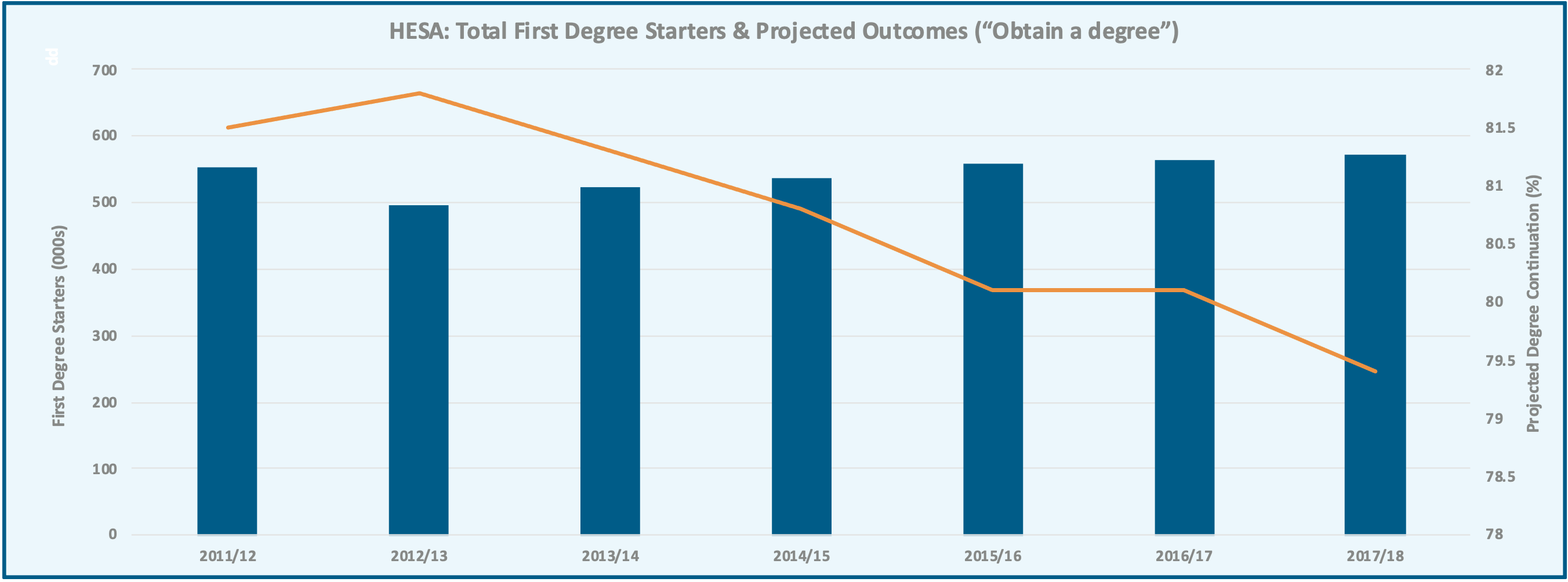
However, pre Covid, there were a number of potential drivers increasing university dropout rates – these included the changing profile of students who may not have had the skills required for a study or not having adequate access to support.
Due to the Covid pandemic, there are different student concerns to consider including increased loneliness, experiencing dissatisfaction, and impaired mental health.
The number of students dropping out of their university courses across the UK has been lower in Summer term 2020 than in previous years – possibly due to the lack of any better alternatives.
Give the right circumstances, Artificial Intelligence can help: early detection of students who are struggling within their studies means early intervention from the university, ultimately decreasing the chance of them dropping out later. AI or machine learning can tap into the data that the university has at its disposal to predict at-risk students.
Roundtable Discussion: Key Points
1. Data is the foundation, however the foundation needs to be much stronger.
Good data is the foundation that is required for effective learning analytics, and for algorithms to be built upon.
However, a number of challenges were identified such as:
- lack of effective management information systems.
- fragmented way of recording why students drop out
- not getting usable feedback from students once they’ve left
There was a need to look at how data is collected before we jump into AI solutions.
2. Covid has raised some unique issues in the context of student success and student retention.
The issues identified are wide ranging:
- Workload: students working on health programmes linked to the NHS – they are facing high workloads which will impact on how they juggle priorities.
- Finance: Covid has increased financial pressure on students – which in turn will have impacted their mental health.
- Mental Health: Students are dropping out because of mental health issues.
- Digital Poverty: Students not being able to access the internet – universities have had to provide support with internet access and laptops.
- Online: An unexpected advantage has been that the move to online has provided better support for a certain segment of students who may have otherwise dropped out.
- Online: Some student segments particularly like the flexibility that online learning provides.
- Safety Net policy: This was designed to ensure that students received at least the degree classification that they were on track to achieve before the Covid situation developed. This had an initial positive impact on student progression, however, some of those who benefited are now starting to struggle.
- Economy: Once the economy picks up, there could be an increase in students dropping out.
3. The application of AI must support a clear objective and must consider the ethics.
There are a set of questions to answer prior to using AI:
- What are we trying to achieve?
- How do we know we’re using the data correctly (especially if it’s insufficient)? What are we going to do with the answers?
- Who makes the decisions, how important are the outcomes from the AI?
- How do we protect against biases? Without proper care in programming AI systems, you could potentially have the bias of the programmer play a part in determining outcomes.
- What’s driving it? Income, success rates/reputation or concern for the student?
4. AI is being used to support student success interventions.
An example discussed:
- Objective: to predict outcomes from the first year (i.e. are students likely to get a First, a 2:1, a 2:2 at the end of the course) such that early interventions could be made.
- Issues and challenges:
- not having a full set of data,
- programmes changed so the models were no longer valid, and
- key data variables (e.g. attendance) which were thought would be relevant turned out not to be
- final year result relied heavily on a final year project.
5. Whatever the university approach or intervention, the student must benefit and see the value (of the approach or intervention).
- One goal is to ensure that the student is on the right programme from the start. There could be a way to use more data from a student’s background (i.e. data from school) to determine whether the student is suitable to go into the programme the student has selected. However, there are ethical issues to consider.
- A learning analytics dashboard is more about the institution surveilling the students. Students probably don’t know that their data is being aggregated in this way.
- Analytics should be focused on gathering information for the students for their own learning – focussing on the individuals and supporting them.
6. The problem definition is critical.
- Define the problem you want to solve, and what a good outcome looks like – we can be seduced by data and packages that promise impressive outputs. The issue may not be with data or the machine learning but with the goal and the success criteria.
- Start with the human problem first – do not start with how we can use this technology – start with what is the need.
7. The Yin and Yang of Humans and Technology needs to evolve.
- People and data/technology need to work together – there is a lack of understanding what data/AI can do – AI is more limited than people imagine.
- We also need any AI applications for student success to have student involvement in the design i.e. students as partners or co-designers.
- There is a technology fallacy which revolves around the mistaken belief that because business challenges are caused by evolving digital technology innovations, the solutions are likely to come from digital technologies. Kane et al. (2019) suggests that we need to shift our focus from technology to people when investigating digital transformations.
8. The pace of change in universities can be slow, so cases for change and investment need to be compelling.
- Infrastructure and operational investments will cost money, so the benefits need to be clearly articulated in a compelling way to senior university teams.
Hopefully you’ve found this article interesting. If you would like to discuss this roundtable topic or would like to attend our next Higher Education and AI roundtable, please contact us.
Established in 2012, Whitecap Consulting is a regional strategy consultancy headquartered in Leeds, with offices in Manchester, Milton Keynes, Bristol and Newcastle. We typically work with boards, executives and investors of predominantly mid-sized organisations with a turnover of c£10m-£300m, helping clients analyse, develop and implement growth strategies. Also, we work with clients across a range of sectors including Financial Services, Technology, FinTech, Outsourcing, Consumer and Retail, Property, Healthcare, Higher Education and Professional Services, and Corporate Finance and PE.